Does this machine learning model predict large earthquakes? Maybe not.
We examine a new earthquake paper in Nature Communications.
Citation: Bradley, K., Hubbard, J., 2024. Does this machine learning model predict large earthquakes? Maybe not. Earthquake Insights, https://doi.org/10.62481/e64960d4
Earthquake Insights is an ad-free newsletter written by two earthquake scientists. Our posts are written for a general audience, with some advanced science thrown in! To get these posts delivered by email, become a free subscriber. If you would like to support our work here, please also consider a paid subscription.
Girona and Drymoni (2024) build a machine learning model that apparently sees hidden earthquake signals during the weeks to months before two large (M7+) earthquakes in Alaska and California. They propose that their method can identify when the regional fault system is preparing for a big earthquake. If they are correct, then this is a landmark advance in large earthquake forecasting.
But does the study hold up to scrutiny? We replicated their results, and then further tested the method. Briefly, it appears that the prediction of the earthquake in Alaska is due to faulty data in the test catalog, and disappears when the most updated USGS catalog is used. The prediction of the earthquake in California appears to arise from natural volcanic earthquake swarms in the Coso Volcanic Field.
When we tested the published model against fifteen additional large (M6.2+) earthquakes in Alaska and California, we found that none of them showed any precursory unrest signal.
We conclude that the model simply does not work, and that the study does not provide any new evidence for abnormal low-magnitude seismicity prior to large earthquakes.
A quick note: This post represents our best reading and analysis of this paper. If we have made any mistakes, please let us know and we will correct them immediately. All of our scientific paper analyses always remain free to read and open to comment.
On August 28, 2024 a paper titled “Abnormal low-magnitude seismicity preceding large-magnitude earthquakes” was published in Nature Communications, authored by Dr. Társilo Girona and Dr. Kyriaki Drymoni.
The paper (henceforth referred to as GD2024) makes a striking claim about earthquake forecasting:
In this manuscript, we demonstrate that […] low-magnitude seismicity can alert about impending large-magnitude earthquakes in Southern California and Southcentral Alaska. - GD2024
Seismologists have long searched for signals that give warning of upcoming large earthquakes. Despite efforts spanning more than a century, no such system has ultimately worked. If such a signal could be found in the most widely and cheaply collected earthquake data (catalogs of previous earthquakes, recording location, magnitude, and time), the importance of its discovery would be hard to overstate.
When papers like this are published, the media coverage can be a bit breathless.
“AI revolutionizes earthquake prediction.” - Shubham Arora for TimesNowNews.com
“UAF scientist’s method could give months’ warning of major earthquakes.” - Rod Boyce at the Geophysical Institute, University of Alaska Fairbanks
As we stand on the brink of a new chapter in earthquake forecasting, propelled by the fusion of machine learning and seismic research, the potential for preemptive warnings of major seismic events offers hope for saving lives and mitigating economic losses. - Tyler O’Neal for SuperComputingOnline.com
To be fair to the authors, media narratives can easily go out of control when earthquakes are concerned.
To be fair to the media, the authors do directly suggest that their machine learning method will change the practice of earthquake forecasting:
Eventually, our approach could help to design earthquake alert level strategies based on the detection of regional tectonic unrest, and to improve the forecast of large-magnitude earthquakes from weeks to months in advance. - GD2024
In this post, we will take a close look at the study, so we can determine for ourselves how reliable the results are. We will try to keep the technical details to a minimum, focusing on simple tests using the provided code and explaining how the results of the tests affect the reliability of the paper.
Prediction vs. forecast: what’s the difference?
GD2024 describe their method as a forecasting, and not a prediction, technique — although they use the word “prediction” eight times in the paper.
What is the difference between earthquake forecasting (a noble endeavor untarnished by time) and earthquake prediction (a scurrilous endeavor with a deservedly bad reputation)?
An earthquake prediction is a proposal of the timing, location, and magnitude of one future earthquake. Predictions can be very specific: “A magnitude 7.42 earthquake will occur 2 kilometers east of Whoville on March 12, 2083,” or more generic: “A magnitude 6.5+ earthquake will occur somewhere in Whatsitland between January 2026 and January 2036.” Virtually all earthquake predictions involve large earthquakes of societal relevance.
Earthquake predictions are falsifiable. After the time window has passed, the predicted earthquake either did happen, or it did not. The typical approach taken by earthquake predictors is to trumpet any successes and ignore any failures.
As earthquake predictions become more generic, incorporating probabilities for each aspect, they morph into earthquake forecasts. This is an example of a forecast: “There is a 10% probability that a magnitude 6+ earthquake will strike in Southern Wherever during the next five years.” Due to their probabilistic nature, forecasts can never really be falsified. Only after a long period of forecasting can we compare the statistics of actual events to their previously estimated probabilities.
The easiest way to tell whether a scientific study is talking about prediction or forecasting, regardless of the terminology used in that study, is to look at how earthquakes are actually treated.
Studies that propose an earthquake prediction method will usually look at only a few previous large earthquakes. They will attempt to show how we could have seen these earthquakes coming, if only we had been monitoring this or that information. The implication is that there are specific signs that a large earthquake is approaching. If we watch out for these signs, we can raise a red flag when they happen, effectively predicting an impending earthquake.
Some, but not all, earthquake prediction studies will incorporate non-tectonic data, like solar flare activity, celestial alignments, animal freak-outs, weather patterns, etc. They can do this because they only need to fit the oddball data to a few specific large earthquake occurrences. The estimated probabilities of the large earthquakes in these studies will always be high. After all, a model that produced only low probabilities for large earthquakes that actually did happen would not be kept in production for long.
In contrast, earthquake forecasting studies will not typically focus on individual earthquakes, because only the broad statistics are of interest. These studies will generally look at entire catalogs of earthquakes, combining the location, magnitude, and timing of all of the events to produce a space-and-time map of estimated probabilities of future earthquakes of all magnitudes — not only the largest. They might also include information on how fast faults are slipping, or how much the crust is deforming in general. However, earthquake forecasting methods rarely include non-tectonic information because those data can never outweigh the observed occurrence rate of the actual earthquakes. The probabilities of large earthquakes in these forecasts are always low — perhaps a few percent per year — matching the basic fact that large earthquakes are always rare. A great example of a forecasting approach is the new National Seismic Hazard Model covering the entire United States.
So, is GD2024 actually a forecasting paper, or is it a prediction paper?
GD2024 examines earthquake behavior leading up to only two large (well, kind-of-large) earthquakes: the 2018 M7.1 Anchorage, Alaska earthquake, and the 2019 M7.1 Ridgecrest, California earthquake (technically, its M6.4 foreshock). In their method, for each moment in time, the authors estimate the probability of an impending large earthquake (Pun, in percent chance over the next 10 days) at the epicentral locations of those two earthquakes. They show that, for these two earthquakes, their estimated Pun value rose to high levels (20-40%) over the months before the actual earthquakes, but never rose that high over the 3 years prior.
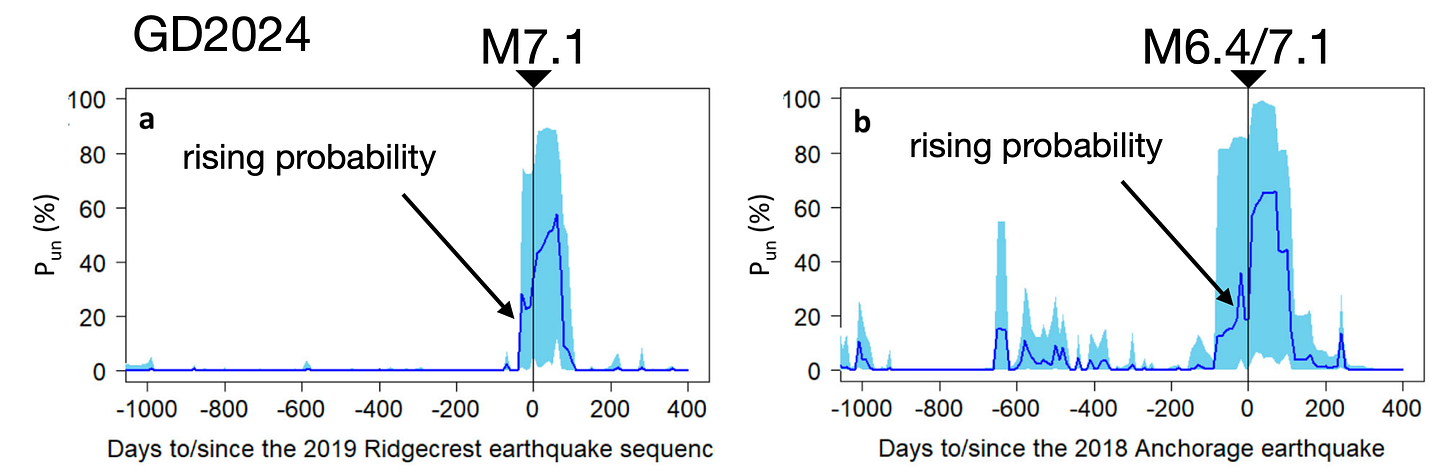
The implication is that their model can see large earthquakes coming, using only seismic catalog data as input. The sudden precursory uptick in Pun is supposed to be read as a prediction of an upcoming large earthquake. Note how this method does not produce systematic probabilities for earthquake populations, but is only tested against two specific large earthquakes. By our above criteria, GD2024 is clearly an earthquake prediction paper, and the method is intended to be used to predict upcoming events with months of lead time.
One model out of many
Before we start our replication, we want to highlight a major issue with the GD2024 study. They didn’t build one model; they built twenty — using different seismic catalogs and data selection parameters to train twenty difference machine learning models. In these different scenarios, GD2024 used different data selection radii, different unrest periods, and different ranges of included earthquake magnitudes — variables that collectively are described as hyperparameters. The important thing is to understand that they looked at the performance of all of those models (which they call scenarios), and then decided which one they liked best — that is the single model presented in the paper.
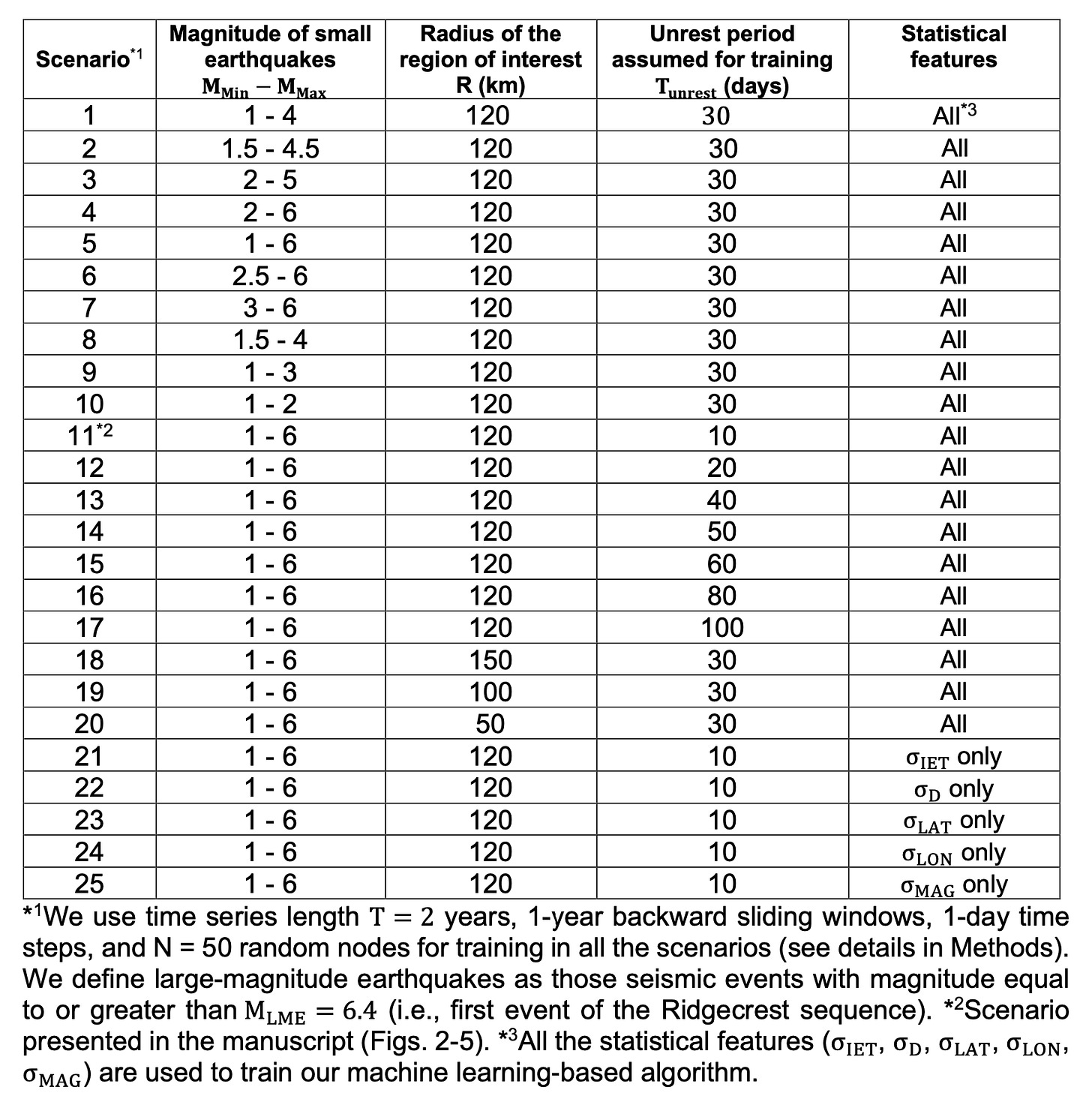
If most of these model scenarios perform fairly well, then selection of a single model out of many isn’t really an issue. However, if the model performance is not great in most of the scenarios, then it is much more likely that the selected model only works by chance.
How do the various scenarios perform? The authors present their results in the supplement. Here, we’ve combined their figures, cutting out the irrelevant time period after the earthquakes. To decide if a model worked well or not, we had to choose our own “prediction criterion.” We’ve arbitrarily decided that to count a result as a successful prediction, Pun must reach at least 30% during the unrest period used in the model.
In the figure below, we have drawn a horizontal orange line at Pun = 30%. We added stars to show whether Pun did exceed 30% just before the earthquake (a green star) or not (a red star). We also added labels for false positives (FP) — i.e., times when the probability went up above 30% but no earthquake happened. Red stars and FP labels are bad. Green stars are good. Scenario 11, the scenario discussed in the main text, is surrounded by a black box.
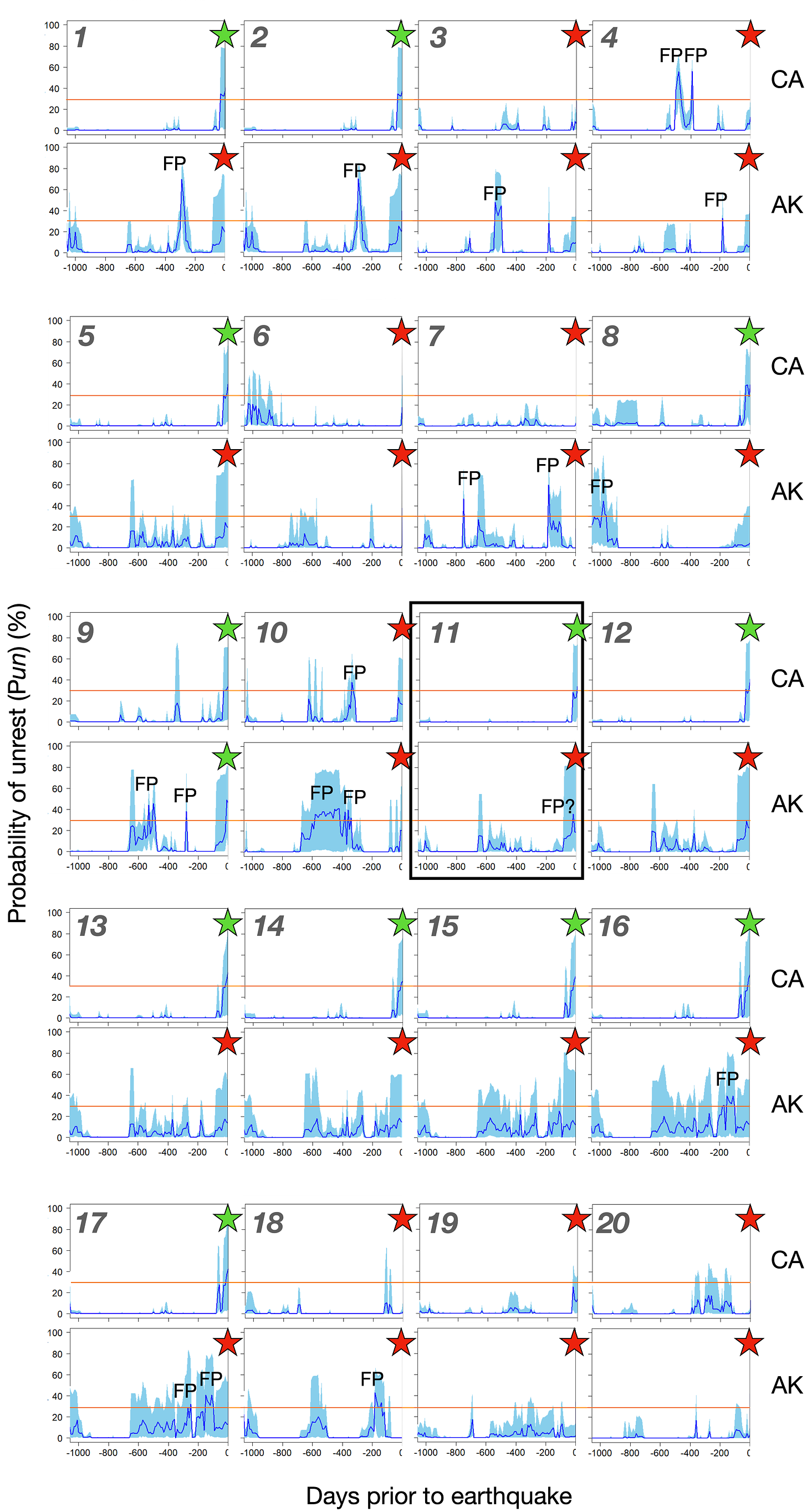
A scenario only needs to accomplish two things to be successful: it doesn’t predict non-existent earthquakes (it avoids false positives), and it successfully does predict the actual earthquakes (it avoids false negatives).
Interestingly, none of the scenarios actually meet our criteria — not even scenario 11.
Only Scenario 9 successfully predicts both earthquakes — but it also contains several false positives. Scenario 11, which is highlighted by the authors in the paper, successfully predicts the Ridgecrest earthquake technically not the Anchorage earthquake. There is a technically a false positive shortly prior to the earthquake, but outside of the 10-day unrest window considered in that scenario.
Since there are no strict definitions provided for what constitutes a successful prediction, the authors can reasonably count Scenario 11 as successful. Ultimately, GD2024 propose Scenario 11 as a robust earthquake prediction scheme. On the topic of the other scenarios, they write:
The parametric analysis reveals that … Our results are little sensitive to the hyperparameters, thus demonstrating the result of the method. - GD2024
This is surprising to us, because when we examine the results of all of the scenarios, it is clear to us that the modeling is very sensitive to the choice of hyperparameters. The output predictions vary wildly between scenarios, even when the hyperparameters don’t seem to change that much.
The selection of a single best-performing model from among many candidates raises a critical issue around the distinction between training data and test data. Machine learning models like the one used in this study are black boxes: we’re not expected to understand what the computer is doing; it’s not based in geology or physics or any form of human logic. So it is critical to test the results of the machine learning algorithm to prove that the computer has learned something real.
To do this, the machine learning method relies on the strict separation of training and test datasets. The complete independence of these data is critical because the only way to evaluate the usefulness of the model is its success in predicting the test data.
But when the method involves generating many models by tweaking the hyperparameters, and then comparing the results for the different test data, there is a risk that the selected model only performed well due to chance, or due to variations in the data unrelated to the process being studied.
By tweaking the hyperparameters, the test data are effectively being used as training data. This can be a useful step in building a machine learning algorithm — but it means that the process is not over. After a hyper-parameter search has been performed, it is necessary to re-run the selected model against a completely independent test dataset. That will ensure that the model wasn’t simply tuned to fit the test data.
So, our strategy in this post is to first try to replicate the actual results of the paper, and then test the published models against more earthquakes.
Phase 1: Replicating the main results of the paper
We started our analysis by replicating the main results of the published study, using the code and data provided. We were able to create all of the new figures using the code directly, with only very minor modifications like setting paths to data files on our own computer.
First, we recalculated the Scenario 11 times series presented as Figure 2 of BD2024, which are the primary support for the proposed earthquake prediction scheme. This is a simple check to make sure that the models and the test data can reproduce the figures in the paper. The published models are at left, and our replications are at right (marked BH2024).
We achieve an exact match, which tells us that the code is working as intended.
Our next step was to examine the training and test datasets. GD2024 employ a machine learning method, which uses a training catalog of earthquakes to build a model. This model is then tested against a later, independent earthquake catalog from the same area. Validation of the training and test data is an important step that is often skipped during peer review.
We quickly noticed that the training data provided for download (the USGS earthquake catalog, for events larger than magnitude 1, from 1989 through 2012) was incomplete for both Alaska and California, as shown on the timelines below.
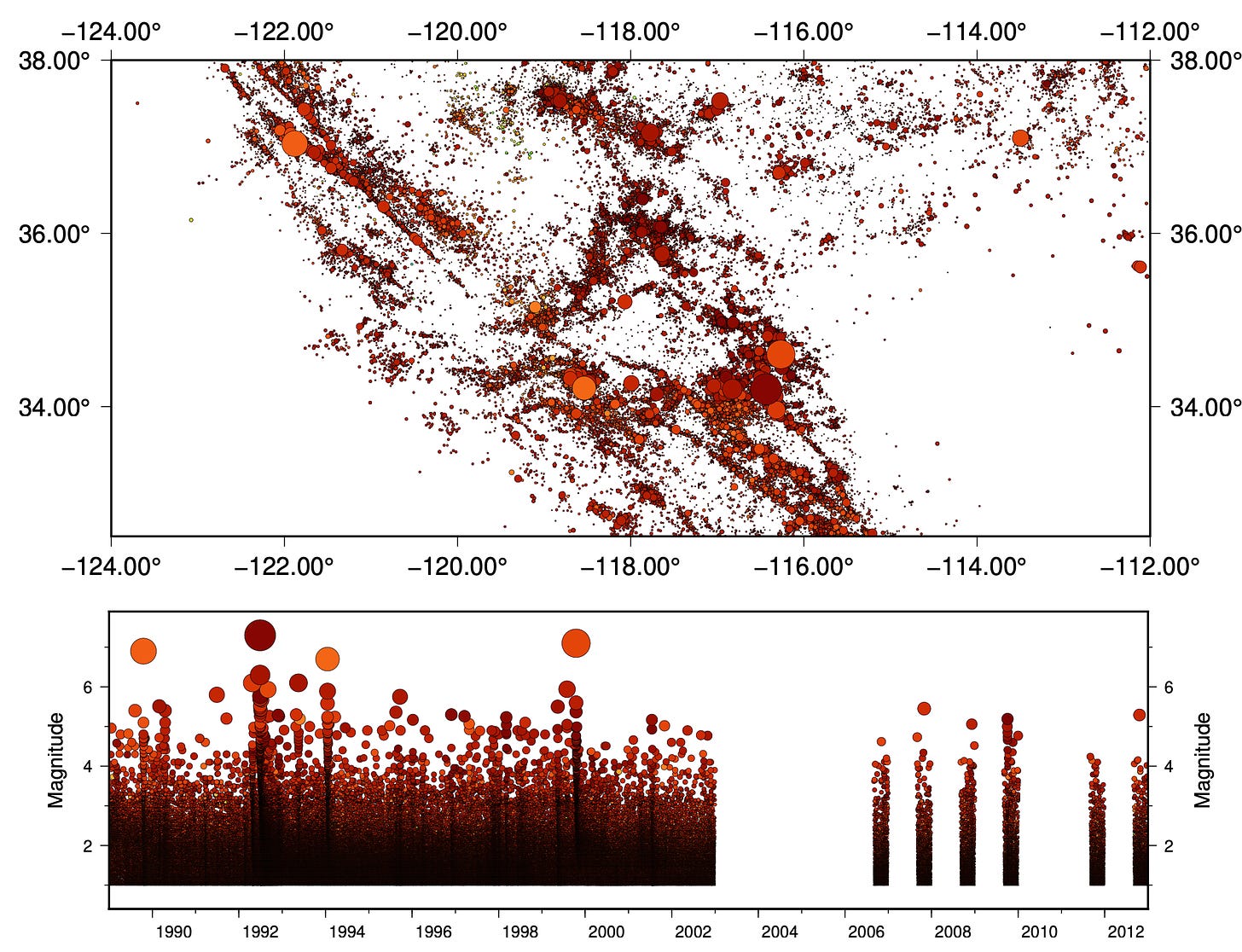
This initially worried us, because we thought that the models might have been trained against incomplete data. Fortunately, by examining the published model files directly, we eventually verified that they were in fact trained on a complete dataset. The missing training data seems to be a simple error in copying into the online data folder shared by the authors.
We decided to re-download the earthquake data ourselves in order to have complete training and test data. This study uses the USGS (ANSS) earthquake catalog, which is freely downloadable. We downloaded the earthquake catalog (magnitude >=1) for both study areas for the training and test periods. The download was performed on September 11, 2024.
We then re-ran Scenario 11 using the published models and the updated test data. Surprisingly, the results were not the same as the publication:
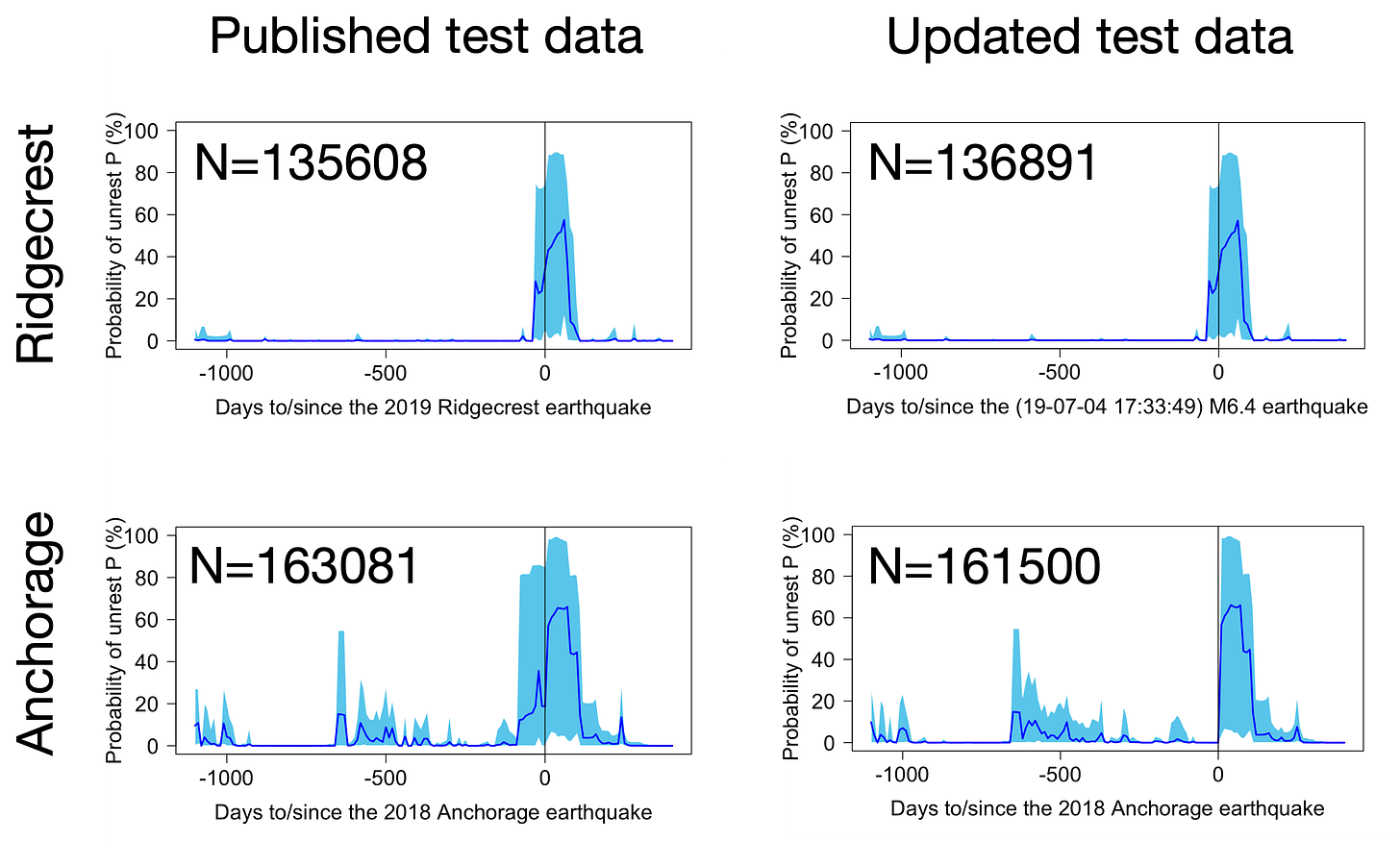
While the Ridgecrest earthquake results are very similar, the Anchorage earthquake is not predicted using our updated test data! Pun only increases after the M7.1 earthquake actually happens. This is not related to the missing training data (training data are from 1989-2012) — instead, there is a difference in the test data (the time periods around the 2018 Anchorage and 2019 Ridgecrest earthquakes).
So, what’s different? The first thing we noticed is that the number of earthquakes (N) is not the same in the original and updated catalogs. Our updated test dataset for Alaska has ~1500 fewer events than the original dataset. These different events are apparently responsible for the early prediction of the Anchorage earthquake in GD2024.
We weaseled out which earthquakes are present in the published test catalog but not our updated catalog; the map and timeline below show only those events. While the map shows some patterns, the really interesting information is in the time series: the earthquakes are projected to the right to show when they happened.
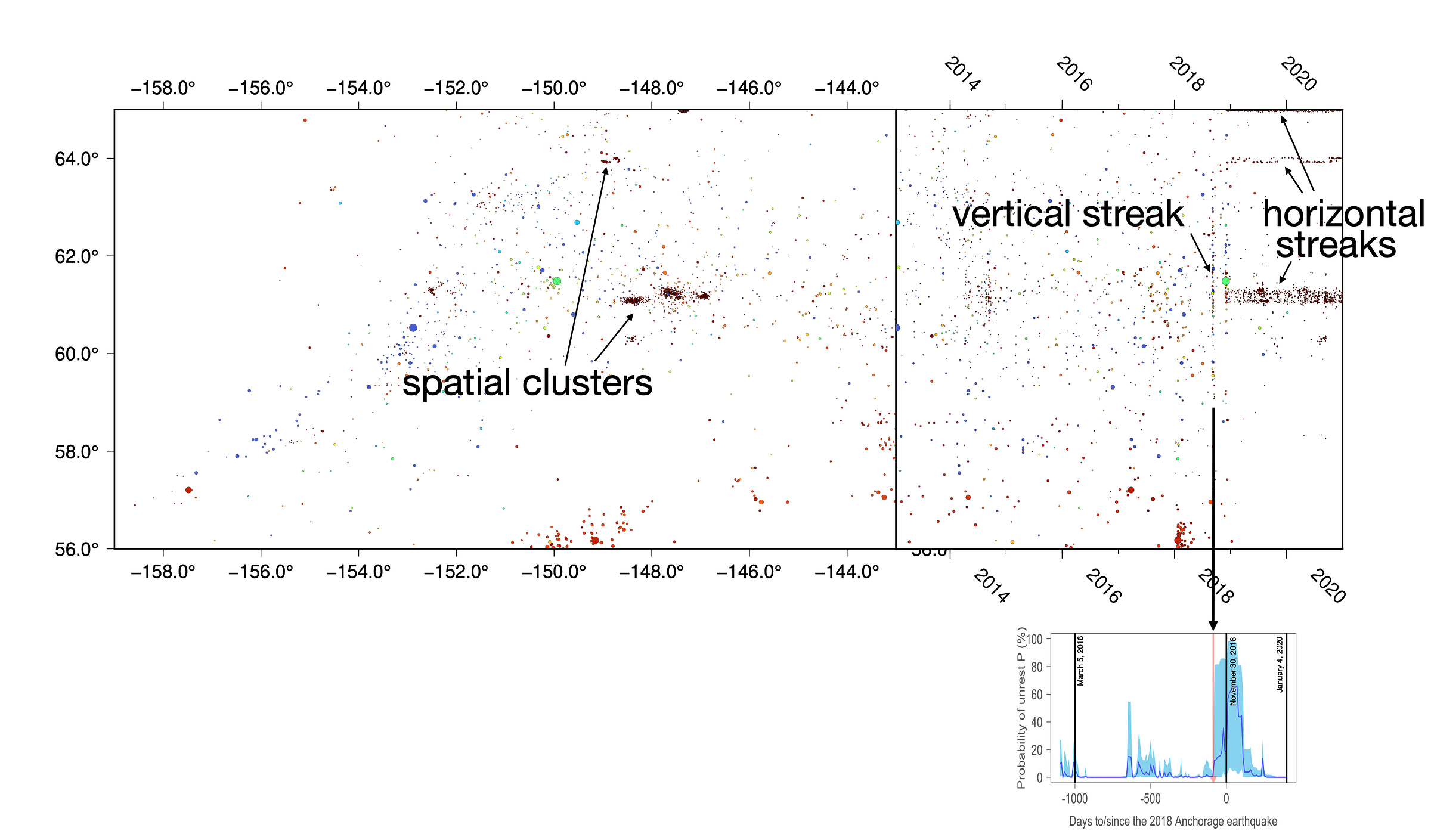
The first thing to note is the vertical streak on the timeline in early September 2018. For some reason, there are an anomalous number of events over a ~10 day period, scattered across Alaska. These events are tectonic earthquakes that were included in the USGS catalog when the published test dataset was downloaded by GD2024, but not when we downloaded the data on September 11. Interestingly, this vertical streak of data appears to match up exactly with the first precursory increase of Pun.
Examination of the event IDs on the USGS website reveals that these events have been deleted from the current USGS database. We don’t have these events in our more recently downloaded catalog because, at some point, they were deleted (no reason for deletions of events is usually given).
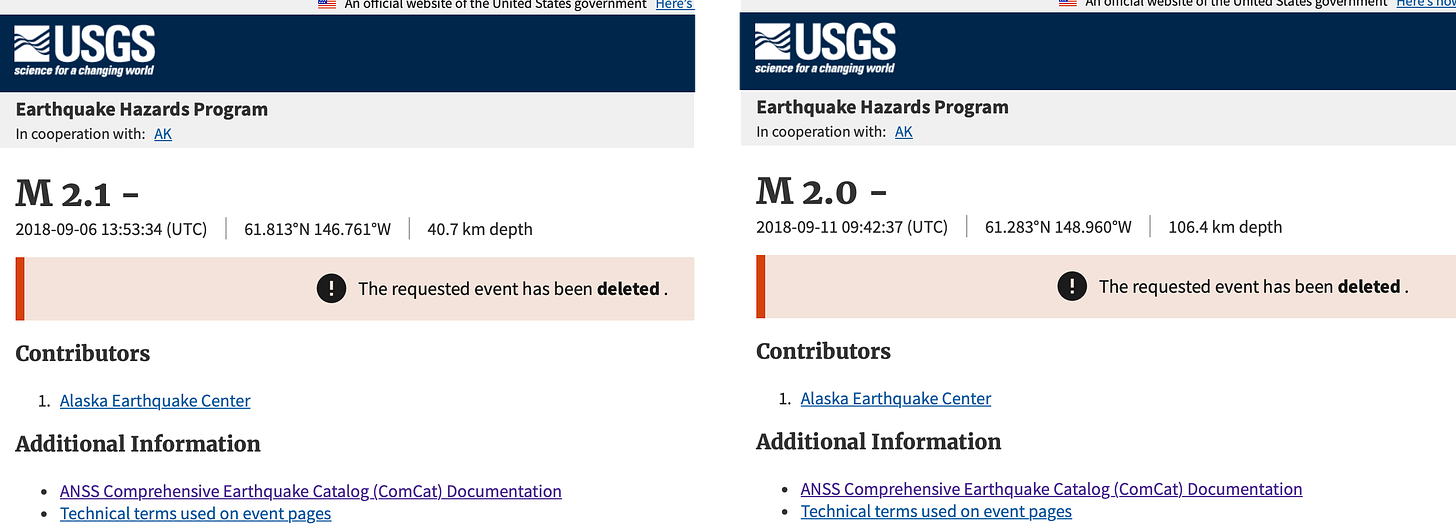
Figure 8 also shows a clear horizontal streaking on the timeline after the Anchorage earthquake. These events come in clusters of shallow earthquakes and are clearly caused by human activity — they are anthropogenic. We checked the published test datafile and found that these earthquakes are labelled as explosions and quarry blasts — events that are usually removed from earthquake catalogs (and which are not present in the training datasets — we checked). Similar events exist in the published test catalog for California, for a similar time period. The appearance of anthropogenic events in the later part of the test catalogs suggests that the catalogs were composited from data downloaded at different times with different query parameters, and probably several years ago.
Earthquake catalogs are dynamic things. As data or processing errors are discovered, errant events can be deleted or updated. As new data are ingested, events can be added back in. There is a significant delay in processing of some earthquakes in the ANSS (USGS) catalog, because multiple different offices contribute detections to the overall catalog, and their contributions must be reviewed. This can lead to delays of up to six weeks for finalization of recent events. It is not surprising that a published earthquake catalog will be slightly different from the most updated version.
Ultimately, we can’t replicate the Anchorage earthquake prediction with a newly downloaded catalog, and we can’t explain exactly why the published test catalog differs from the one available today.
Phase 2: Testing the model against more earthquakes near Alaska
The GD2024 model was only tested against two candidate earthquakes: the 2019 Ridgecrest and 2018 Anchorage earthquakes. It is reasonable to expect that the model should also apply to any earthquake that would have been targeted in the training phase; the machine learning model was trained using data from M6.4+ earthquakes with at least three years of prior data.
While the California study area simply had no other large earthquakes (aside from Ridgecrest) during the test period, the Alaska test dataset does contain a number of events that would have qualified as target events for the training algorithm. Thus, they also are appropriate test events.
Here are the results of those tests. The charts show the value of Pun for the 100 days surrounding each earthquake, using the published models and the updated test catalog. As you can see, the predictions fail for all of the earthquakes (including the 2018 M7.1 Anchorage earthquake, as discussed above). The 2018-01-23 earthquake is located near the edge of the region and therefore might not be a valid target. We will put these results onto a map in a moment.
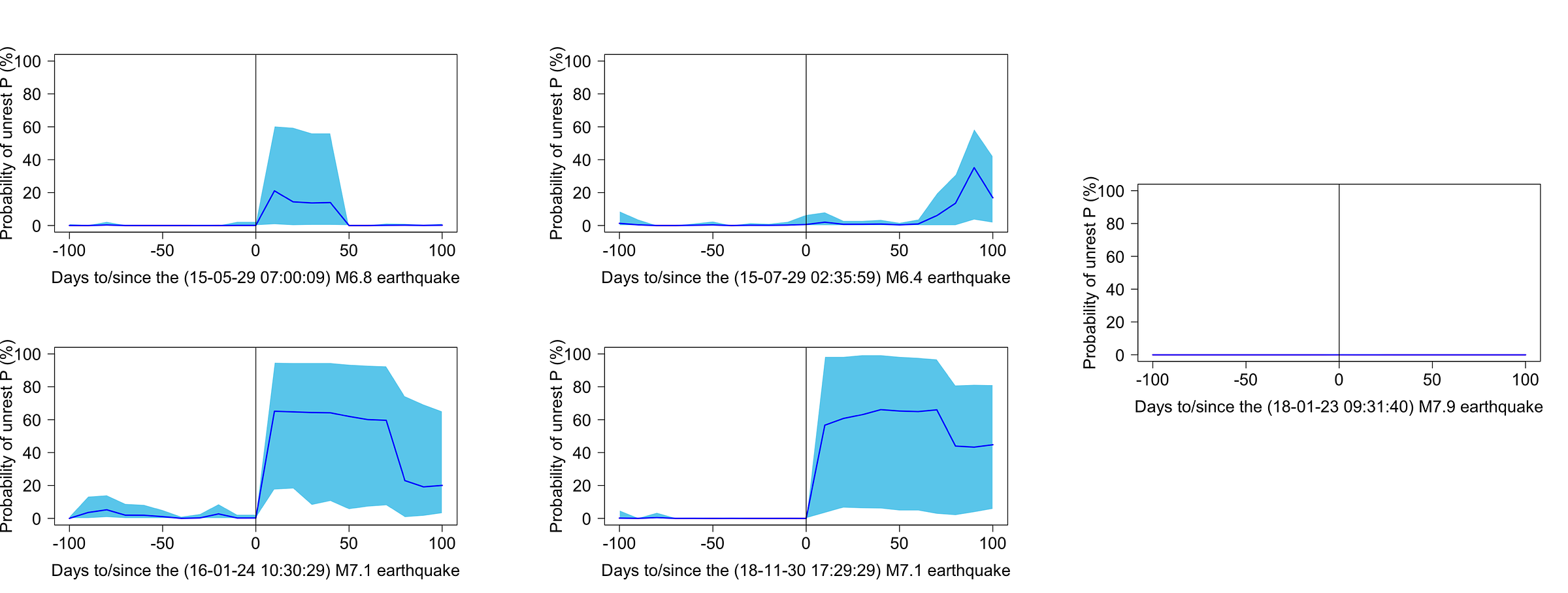
Can we find more earthquakes to test? It is easy to extend the test region slightly (4° in each direction), and also update the catalog through September 2024. This gives us access to six more nearby M6.4+ earthquakes, with the latest happening in 2023. Some of these events are quite geologically similar to the training target earthquakes. We plotted the results from these earthquakes, and the previously discussed earthquakes, on this map:
As you can see, when we test all 11 of the M6.4+ events that happened between 2015 and 2023, there are no cases of successful prediction by the published model. This includes the M7.1 Anchorage earthquake. The only two events that do show a high probability of unrest before the upcoming earthquake are simply showing the lingering effects of aftershocks of earlier, nearby large earthquakes (M7.2, M8.2 - those earlier events are marked in pink on the relevant time series).
We can safely conclude that the published model really just doesn’t seem to work in Alaska, for any earthquake at all — when an updated catalog is used.
Phase 3: Testing the model for more earthquakes near California
We also looked at an extended test area and time window for California. We widened the search area by 4° in each direction, extended the catalog time to September 2024, and lowered the magnitude of potential test events to M6.2+. This gave us an additional five earthquakes. Out of six earthquakes in California, only one exhibits a predictive probability increase: the Ridgecrest earthquake, as shown in GD2024.
Examining the Ridgecrest prediction
The Ridgecrest prediction appears to be robust to catalog updates, but we are wary of studies that focus on this event. In a previous post, we examined a paper that proposed that the Ridgecrest earthquake was heralded by unusual tidal patterns in small-magnitude earthquakes. In that case, we thought that any tectonic signal proposed in that study was probably contaminated by non-tectonic earthquake activity in the nearby Coso Volcanic Field, which is located very close to the Ridgecrest rupture area. The Coso Volcanic Field exhibits unusual seismicity, including natural swarms and anthropogenic seismicity related to active geothermal energy production.
Does seismicity from the Coso Volcanic Field also affect the predictions in this new study? Because the model of GD2024 is a machine-learning algorithm, we can’t intuitively understand how the calculations treat specific earthquakes. However, we can test the impact of these events by removing clusters of earthquakes that are clearly associated with the volcanic field, and then checking whether the model still predicts the Ridgecrest earthquake. If the prediction vanishes when earthquakes are removed, then those earthquakes are at least partly responsible for the prediction. Because these are not tectonic earthquakes, the interpretation of GD2024 of a critically stressed regional fault system would be harder to support.
First, we replicated the prediction of the Ridgecrest earthquake using the original test data, and also with our updated catalog. This time, we reduced the time step from 10 days to 1 day, and focused only on the 50 day period leading up to the earthquake. Each point still represents the probability of a large event over the following 10 days. The zoomed-in graphs give us a better view of when the unrest actually begins (in the model).
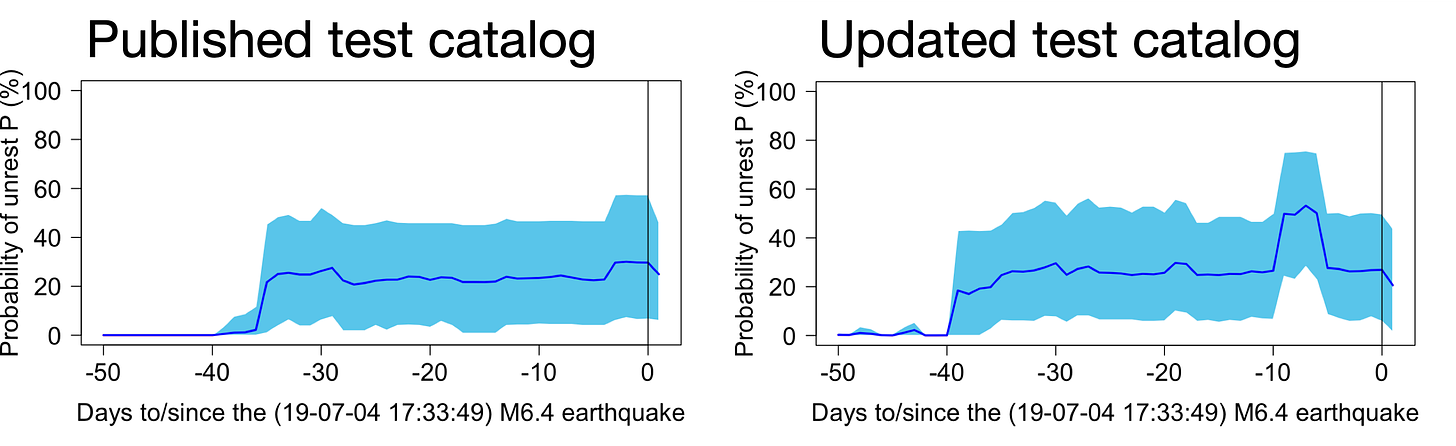
The results are basically the same for both catalogs, with a sudden increase in the probability of a large earthquake, starting between 40 and 37 days before the Ridgecrest earthquake. The updated catalog (downloaded September 2024) has a slightly longer period of unrest, and a brief period of even higher Pun.
Next, we needed to figure out which Coso events to exclude. This is an arbitrary decision, that must be guided by geological judgment. We plotted a timeline of the seismicity within the 120 km radius of the Ridgecrest earthquake, from June 3, 2018 to just before the M6.4 foreshock.
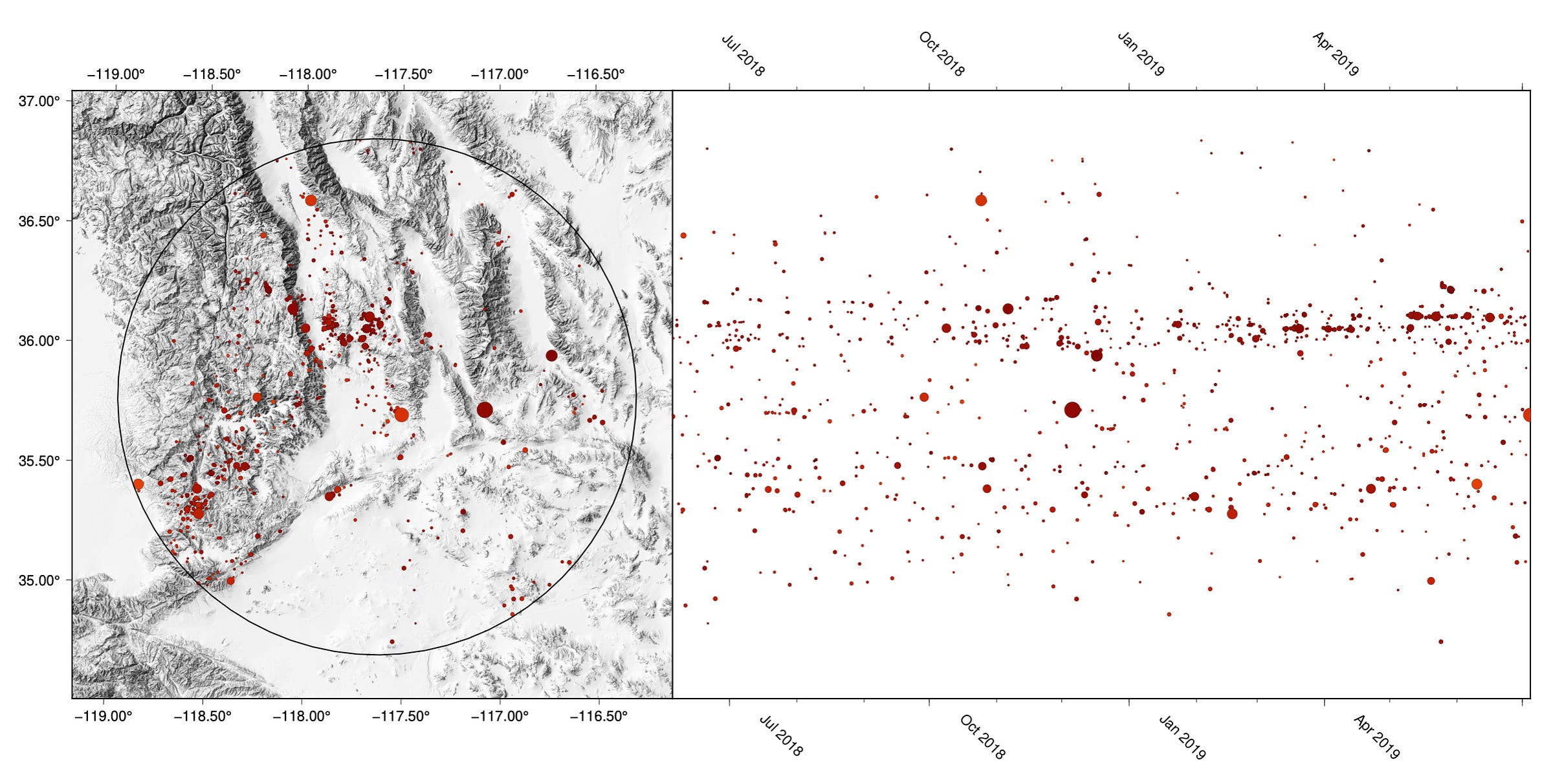
There are clearly some very active earthquake clusters, which don’t move in space but vary in seismic productivity over time. They appear as horizontal streaks on the timeline. Because these clusters don’t begin with large events, they are not simple mainshock-aftershock earthquake sequences. Instead, they are swarms — typical of volcanic and geothermal areas, where hot and pressurized fluids (or even magma movements) in the shallow crust can drive faults to rupture repeatedly in small events, without ever producing large earthquakes.
We removed the earthquakes from the small area of the most productive clusters, and re-ran the calculation on this modified catalog. This is what the timeline looks like after chopping out the swarmiest area:
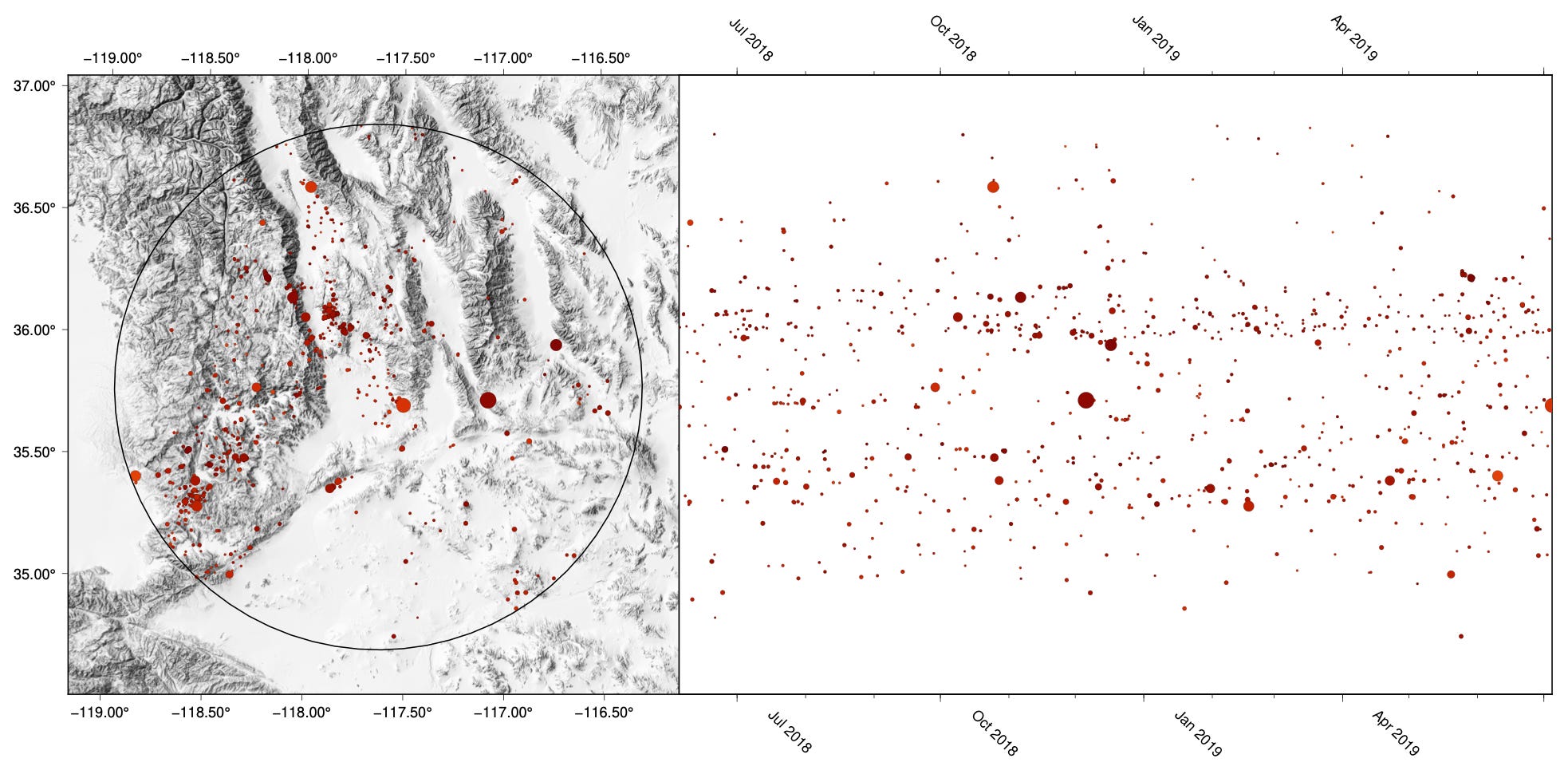
The most active swarms have been removed. The area we chopped out is shown in the inset on the timeline below.
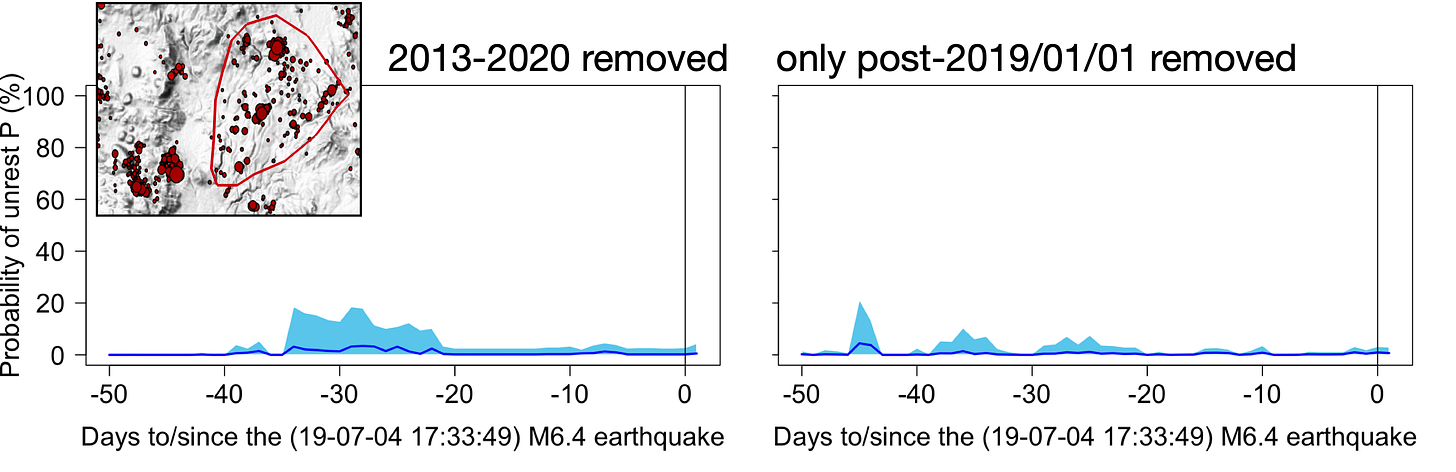
The published model no longer predicts the Ridgecrest earthquake for this modified catalog (left-hand graph). If we remove only the events that happened in 2019, leaving all of the older events in place, the prediction still disappears (right-hand graph). This suggests to us that the volcanic swarms of early 2019 are actually controlling the Ridgecrest earthquake prediction.
We also examined the swarm-like seismicity just west of the previous test case, which is closely associated with geothermal power production at the Coso power plant. These swarms are not as productive, and might be partially due to plant operations.
Interestingly, removal of these events actually extends the period of high earthquake probability to almost three months, more than doubling the period of higher Pun.
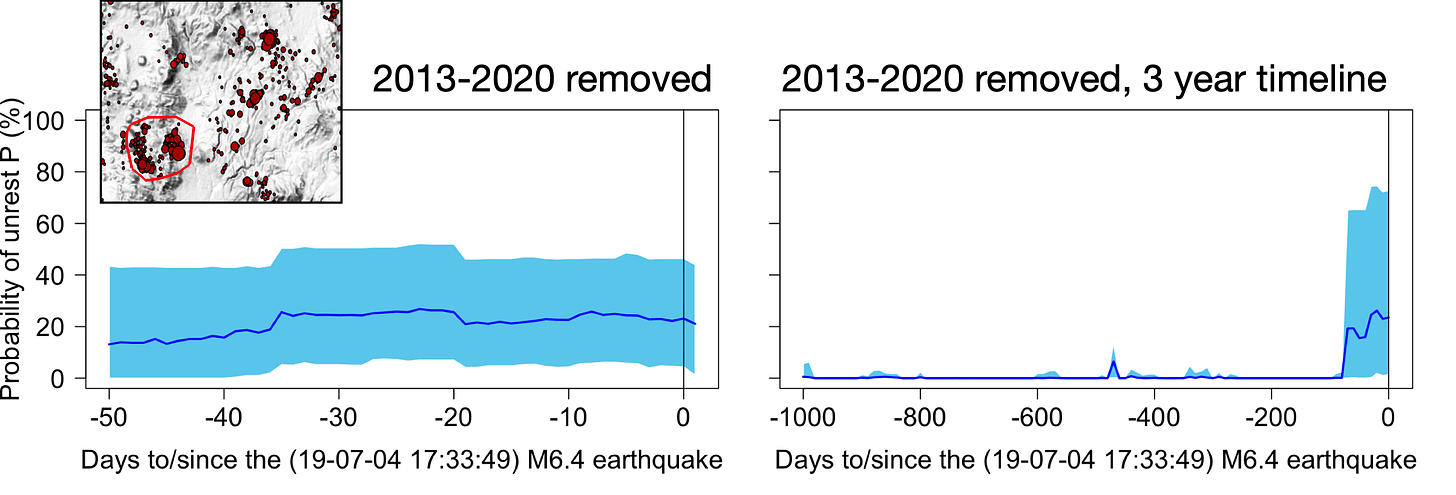
Recall that the model is intended to detect elevated unrest in the ten days prior to a large earthquake — that is how Scenario 11 was trained. Elevated unrest signals outside of that window are not meaningful, or should actually count as false positives: if the machine learning method is working as intended, it should be classifying these periods as low risk. It is clear that swarms, both natural and anthropogenic, are able to create or suppress Pun signals, at various times and without any evidence of a physical connection to the later earthquake.
Ultimately, it appears that the Ridgecrest earthquake “prediction” is intimately tied up with swarm-like seismicity within the Coso Volcanic Field. This is not surprising to us, as it is a continuing issue with papers that uncritically use background seismicity prior to the Ridgecrest sequence.
While it is possible (let’s say, not impossible) that there is some causative connection between the volcanic swarms and the Ridgecrest earthquake, the machine learning model used in GD2024 is particularly ill-suited to studying a possible relationship. We leave a more detailed dissection of that as an exercise to the reader — but we don’t think it is likely to be fruitful!
Summing up
While we verified that the results presented in GD2024 are replicable using the provided models and catalog, we simply cannot get the method to work for updated data or for any other earthquakes in the study regions. It appears that the more optimistic view of the method presented in the paper reflects selection of one specific model parameterization based on its performance against two earthquakes.
It is not surprising to us that a simple machine learning model applied to earthquake catalog data does not effectively predict large earthquakes. The experience of decades of research, much of it highly technical and done by people with great experience in earthquake behavior, is that the solution to earthquake prediction can’t be that simple — if it exists at all. Geological complexity is real, earthquake interactions are real, and a few years of training data collected before a few earthquakes are unlikely to capture this complexity.
Could machine learning methods eventually detect hidden but reliably predictive patterns in earthquake catalogs? That apparently still remains to be seen.
Data and code:
The results presented in our post can be directly replicated using the code provided by the authors with only minor modifications (changing time step variables, etc).
We would like thank the authors for providing their code and data in an online supplement. Without this information, our evaluation of the study would be impossible. While complex methods can be described perfectly in papers, the effort of implementation and testing is simply too high without provided code and data. Whether we agree or disagree about the implications of the paper, it is important that the authors be recognized for their clear commitment to open science.
References:
Bradley, K., Hubbard, J., 2023. Do Earth tides warn of impending large earthquakes? Earthquake Insights, https://doi.org/10.62481/695e5945
Girona, T. and Drymoni, K., 2024. Abnormal low-magnitude seismicity preceding large-magnitude earthquakes. Nature Communications, 15(1), p.7429. https://doi.org/10.1038/s41467-024-51596-z
Girona, T. and Drymoni, K., 2024. Abnormal low-magnitude seismicity preceding large-magnitude earthquakes — dataset, scripts, machine learning models, and results (1.0). Zenodo, https://doi.org/10.5281/zenodo.13212238.
Petersen, M.D., Shumway, A.M., Powers, P.M., Field, E.H., Moschetti, M.P., Jaiswal, K.S., Milner, K.R., Rezaeian, S., Frankel, A.D., Llenos, A.L. and Michael, A.J., 2024. The 2023 US 50-state national seismic hazard model: Overview and implications. Earthquake Spectra, 40(1), pp.5-88. https://doi.org/10.1177/87552930231215428
This was a great read - a few points stuck out to me, not necessarily particular to this study.
I applaud the authors for making their data and code available. This is the direction that open seismology should be going. This is particularly important when using a real-time earthquake catalog such as the ANSS ComCat catalog.
As you mentioned, ComCat is updated and curated continually, contains event information from many different sources (e.g., regional networks and the USGS), and is, therefore, dynamic. Maybe in the future, there can be better versioning of this catalog, but as it stands, including the earthquake catalog underlying the research is an important step for reproducibility.
This brings up another point: earthquake catalogs (estimates of magnitude and location) can be dynamic for many reasons. A set of seismic stations could go down, changing the network configuration, the underlying algorithms to compute locations and magnitudes may change, the normal duty seismologist who processes the events may have been on leave, retrospective QC may be performed. If research relies on the quality of an earthquake catalog, it may be beneficial to reach out to the data providers to ensure that the catalog is being interpreted correctly. For example, a common mistake is unintentionally mixing magnitude types because a catalog may default report different preferred magnitudes based on an event size. You never know if more attention was spent on cataloging earthquakes surrounding significant events because of special interest.
On an aside, when I read a paper focused on earthquake prediction, there are two things I key into. How many events was the method applied to? We have a robust global earthquake catalog, so my expectation is that a significant approach could be observed on a wide range of earthquakes. 2) How much attention was spent looking at the false positive rate. An EQ prediction is only good if we don't cry wolf.
we discussed a similar topic in a recent paper
https://agupubs.onlinelibrary.wiley.com/doi/full/10.1029/2023JB027337